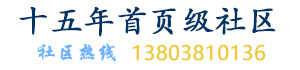
精通
英语
和
开源
,
擅长
开发
与
培训
,
胸怀四海
第一信赖
服务方向
联系方式
1、本文技术和今日头条与抖音技术是类似的,可以根据环境和参数找出类似的内容,当然只是模型,不过也有研究意义。
2、本文充分体现了Python数据处理的优势。
3、复杂算法Python用短短几行就实现了,功能强大。
4、可以学习Python库的一些技巧。
5、本文内容可以是Python机器学习和语音识别模型算法学习的铺垫。
6、需要本文源代码,对本文代码配置,对本文代码有兴趣学习的朋友,请加QQ396806883,锐英源网站是首页级网站就是因为站内太多开源原创内容。
7、要点:功能演示,过滤器预测,用户评分特征算法预测,提取文字特征算法预测
Whenever it comes to data science or machine learning; the first thing that crosses our mind is somewhat prediction, recommendation system or stuff like that. Actually, recommendation systems are pretty common these days. If we talk about some most popular websites like Amazon, ebay, yts and let’s not forget about Facebook, you’ll see those recommendation systems in action. You would definitely have come across some things with the tag ‘you might be interested in’, ‘you might know this person’ or ‘people also searched for’ kind of things. So I decided to take a look at how things work and here I am. We’ll talk about some basic and common types of recommendation systems, how they work and we will develop them using Python. One thing to be noted; these systems does not match the quality, complexity or accuracy used by the tech companies but will just give you the idea and a starting point. 每当涉及数据科学或机器学习时;首先想到的是某种预测,推荐系统或类似的东西。实际上,如今推荐系统非常普遍。如果我们谈论诸如Amazon,ebay,yts之类的一些最受欢迎的网站,并且别忘了Facebook,那么您会发现这些推荐系统正在发挥作用。您肯定会遇到带有“您可能感兴趣”标签,“您可能知道这个人”或“人们也在搜索”这类事物的标签的某些事物。所以我决定看看事情是如何运作的,我现在在这里。我们将讨论一些基本和常见的推荐系统类型,以及它们的工作方式,并使用Python进行开发。需要注意的一件事;这些系统与技术公司所使用的质量,复杂性或准确性不符,只会为您提供构想和起点。
Ipython notebook or now known as Jupyter notebook is one of the most commonly used tech for scientific computation. The main reason for its usage is because it excels in literate programming. In other words, it has the ability to re-run the portion of a program which is convenient when dealing with large datasets. Easiest way to get Jupyter notebook app is installing a scientific python distribution; most common of which is Anaconda. You can download the Anaconda distribution from https://www.anaconda.com/download/ and simply install it using default settings for a single user. Ipython笔记本或现称为Jupyter笔记本是科学计算中最常用的技术之一。使用它的主要原因是因为它在识字编程方面表现出色。换句话说,它具有重新运行程序部分的能力,这在处理大型数据集时很方便。获取Jupyter笔记本应用程序的最简单方法是安装科学的python发行版;其中最常见的是Anaconda。您可以从https://www.anaconda.com/download/下载Anaconda发行版,并使用单个用户的默认设置进行安装。
Our environment is all set up now so let’s actually do something. Create a new folder naming Book Recommendation System (named it this way because we are going to build book recommendation system you can name it anything.) Now launch the anaconda command prompt and start a new notebook by entering the following command: 现在我们的环境已全部设置好,让我们实际做些事情。创建一个新的文件夹命名书推荐系统(之所以这样命名,是因为我们将要建立书推荐系统,因此可以命名为任何东西。)现在启动anaconda命令提示符并通过输入以下命令来启动新笔记本:
$ jupyter notebook
You should see the following screen: 您应该看到以下屏幕:
What it did is create an empty notebook inside our mentioned folder and will also launch a web-based interactive environment for you to work in. Now let’s talk about some commonly used recommendation systems and see things in action. 它要做的是在我们提到的文件夹中创建一个空笔记本,还将启动一个基于Web的交互式环境供您使用。现在,让我们讨论一些常用的推荐系统并了解实际情况。
Note 注意
This article assumes your very basic understanding of working with data science libraries of python. Even if you're new to this, go ahead as I tried breaking down things easy. Also, the dataset I’m going to use for this article is rather a small dataset based on collected data from Amazon and goodreads. You can download it and feel free to experiment. Code will also work fine with any other datasets. 本文假定您对使用python的数据科学库有非常基本的了解。即使您对此并不陌生,也请继续尝试,因为我尝试着轻松分解问题。另外,我将在本文中使用的数据集是一个很小的数据集,该数据集基于从Amazon收集的数据和goodreads。您可以下载它并随时尝试。代码也可以与任何其他数据集一起正常工作。
This is the most basic recommendation system which offers generalized recommendation to every user based on the popularity. But it does make sense even with all the simplicity. Let’s take the scenario of an ice cream parlor. Every other customer orders the chocolate flavor so indeed that is more popular among the customers and is a hit of that ice cream parlor. So if a new customer walks in and ask for the best, he would be suggested to try chocolate flavor. The same is true about tourist attraction, hotel recommendations, movies, books, music, etc. whatever is more popular among the general public, is more likely to be recommended to new customers.
As mentioned before, this type of recommenders make generalized recommendation not personalized. It means that this system will not take into account the ‘personal’ preferences or choices, rather it would tell that this particular thing is liked by most of the users. 这是最基础的推荐系统,它基于受欢迎程度向每个用户提供广义推荐。但是,即使非常简单,它也确实有意义。让我们以一个冰淇淋店为例。每个其他客户都订购巧克力口味,因此确实在客户中更受欢迎,并且受到那个冰淇淋店的欢迎。因此,如果有新顾客走进来,要求最好的话,建议他尝试巧克力味。在旅游景点,酒店推荐,电影,书籍,音乐等方面也是如此。在大众中更受欢迎的是,更有可能被推荐给新顾客。
Building one will clarify the idea behind. Let’s get started. 如前所述,这种类型的推荐器使通用推荐不具有个性化。这意味着该系统不会考虑“个人”偏好或选择,而是会告诉大多数用户喜欢此特定事物。
# In[1]: #importing libraries import pandas as pd import numpy as np
pandas and numpy are two powerful libraries provided by python for scientific computation, data manipulation and data analysis. numpy; above all; provides high performance, multi-dimensional array along with the tools to manipulate it. Whereas pandas is known for its data structures and operations for manipulating data. We will be using both of these libraries in this article. pandas和numpy是python提供的两个功能强大的库,用于科学计算,数据处理和数据分析。 numpy;首先;提供了高性能的多维数组以及用于操作它的工具。pandas以其数据结构和用于处理数据的操作而闻名。我们将在本文中使用这两个库。
# In[2]: #reading the files data = pd.read_csv('listing.csv', encoding = 'latin-1') books = pd.read_csv('books.csv', encoding = 'latin-1')
# In[3]: #using head() function to view first 5 rows for the object based on position. Just to test if we have right data. data.head()
# In[4]: books.head()
# In[5]: # Getting recommendation based on No. Of ratings rating_count = pd.DataFrame(books, columns=['book_id','no_of_ratings']) # Sorting and dropping the duplicates
rating_count.sort_values('no_of_ratings', ascending=False).drop_duplicates().head(10)
# In[6]: # getting the detail of 5 most rated books most_rated_books = pd.DataFrame([4755, 2409, 2194, 4696, 1616], index=np.arange(5), columns=['book_id']) detail = pd.merge(most_rated_books, data, on='book_id') detail
You can also get only the most rated book as follows: 您还可以只获得评分最高的书,如下所示:
# In[7]:
# getting the most rated book
most_rated_book = pd.DataFrame(books, columns=['book_id', 'user_id', 'avg_rating', 'no_of_ratings'])
most_rated_book.max()
# In[8]:
#getting description for most rated book
most_rated_book.describe()
You can also get the description of any column using the same function. 您还可以使用相同的功能获取任何列的描述。
# In[9]:
# description for author
data['author'].describe()
As this is an age of more ‘personalized’ stuff so, popularity based recommenders are not enough to satisfy the need. Thus, there exist Correlation Based Recommenders which would make the recommendations based on the similarity of items (review similarity we’re talking about). The basic idea behind being that if you like this item, you are most probable to like an item similar to it. Correlation Based Recommenders are a simpler form of collaborative filtering based recommenders. They give you more flavor of being personalized as they would recommend the item that is most similar to the item selected before. 由于这个时代是更多“个性化”的东西,基于受欢迎程度的推荐者不足以满足需求。因此,存在基于相关性的推荐器,它们将根据项目的相似性(我们正在谈论的评论相似性)提出建议。背后的基本思想是,如果您喜欢此商品,则最有可能喜欢与该商品相似的商品。基于相关的推荐器是基于协作过滤的推荐器的一种简单形式。他们会给您更多个性化的味道,因为他们会推荐与之前选择的项目最相似的项目。
We are going to use Pearson’s correlation for our recommendation system. This recommendation system would use item based similarity; correlate the items based on user ratings. 我们将在推荐系统中使用皮尔逊相关系数。该推荐系统将使用基于项目的相似性。根据用户评分将项目相关。
# In[1]:
# importing libraries
import pandas as pd
import numpy as np
# In[2]:
# reading files
data = pd.read_csv('listing.csv', encoding = 'latin-1')
books = pd.read_csv('books.csv', encoding = 'latin-1')
# In[3]:
# Checking the data using head function
books.head()
# In[4]:
# calculating the mean
rating = pd.DataFrame(books.groupby('book_id')['no_of_ratings'].mean())
rating.head()
# In[5]:
# getting the description of rating
rating.describe()
# In[6]:
# sorting based on no of ratings that each book got
rating.sort_values('no_of_ratings', ascending=False).head()
# In[7]:
# Preparing data table for analysis
ratings_pivot = pd.pivot_table(data=books, values='user_rating', index='user_id', columns='book_id')
ratings_pivot.head()
As we are interested in finding correlation between two variables, for that, we are going to use Pearson correlation which would simply measure the linear correlation. In this case, we are interested in knowing the relation between two books based on user rating. 由于我们有兴趣寻找两个变量之间的相关性,为此,我们将使用``皮尔逊相关性''(Pearson相关性),该方法将简单地测量线性相关性。在这种情况下,我们有兴趣根据用户评分来了解两本书之间的关系。
# In[8]:
correlation_matrix = user_rating.corr(method='pearson')
correlation_matrix.head(10)
As you can see, now our table contains pearson correlation coefficient values. 如您所见,现在我们的表包含了皮尔逊相关系数值。
# getting the users who rated this particular book (most rated) and making sure rating is not zero
OneManOut_rating = ratings_pivot[4755]
OneManOut_rating[OneManOut_rating>=0]
# In[9]:
# finidng similar books to One Man Out book using Pearson correlation
similar_to_OneManOut = ratings_pivot.corrwith(OneManOut_rating)
corr_OneManOut = pd.DataFrame(similar_to_OneManOut, columns=['PearsonR'])
corr_OneManOut.dropna(inplace=True)
corr_OneManOut.head()
You’ll encounter a runtime warning because of encountering divide by zero. 由于遇到零除,因此您会遇到运行时警告。
But that will not get into our way so it can be ignored. We’ll still get the output as follows: 但这不会进入我们的视线,因此可以忽略。我们仍然会得到如下输出:
# In[10]:
OneManOut_corr_summary = corr_OneManOut.join(rating)
# In[11]:
# getting the most similar book
OneManOut_corr_summary.sort_values('PearsonR', ascending=False).head(10)
# In[12]:
# getting the details for most similar books
book_corr_OneManOut = pd.DataFrame([2629, 493, 4755, 4571, 2900, 1417, 2681, 1676, 2913, 1431],
index = np.arange(10), columns=['book_id'])
summary = pd.merge(book_corr_OneManOut, data,on='book_id')
summary
Now if you see most rated book in our dataset which is One Man Out: Curt Flood Versus Baseball is of law genre but our recommendation engine is giving us mixed recommendations including Travel, Law, etc. This is because we are using the relation between ratings to make our recommendation. This book was rated 4 times in our dataset and so was the very first recommended by our recommendation engine. It means our recommender is working. 现在,如果您在我们的数据集中看到评分最高的图书,那就是“单人出场:马特洪灾与棒球”是法律类型,但是我们的推荐引擎为我们提供了混合建议,包括旅行,法律等。这是因为我们使用的是评分之间的关系提出我们的建议。这本书在我们的数据集中被评为4倍,因此是我们推荐引擎首次推荐的书。这意味着我们的推荐器代码工作正常。
There exists another type of recommender known as content based recommender. This type of recommender uses the description of the item to recommend next most similar item. Content based recommenders also make the ‘personalized’ recommendation. The main difference between correlation based recommender and content based recommender is that the former considers the ‘user behavior’ while later considers the content for making recommendation. Content based recommender uses the product features or keywords used in description to find the similarity between the items. Let’s see how can we build one. 存在另一种类型的推荐器,称为基于内容的推荐器。此类推荐器使用商品说明来推荐下一个最相似的商品。基于内容的推荐者还会提出“个性化”推荐。基于关联的推荐器和基于内容的推荐器之间的主要区别在于,前者考虑“用户行为”,而后者则考虑进行推荐的内容。基于内容的推荐器使用产品功能或描述中使用的关键字来查找项目之间的相似性。让我们看看如何建立一个。
# In[1]:
# importing libraries
import pandas as pd
from sklearn.metrics.pairwise import linear_kernel
from sklearn.feature_extraction.text import TfidfVectorizer
linear_kernel is used to compute the linear kernel between two variables. We would use this function instead of cosine_similarities() because it is faster and as we are also using TF-IDF vectorization, a simple dot product will give us the same cosine similarity score. Now what is TF-IDF vector? We cannot compute the similarity between the given description in the form it is in our dataset. This is practically impossible. For this purpose, Term Frequency-Inverse Document Frequency (TF-IDF) is calculated for all the documents which would simply return you a matrix with each word representing a column. sklearn’s TfidfVectorizer would do this for us in a couple of lines: linear_kernel用于计算两个变量之间的线性核。我们将使用此函数代替cosine_similarities(),因为它更快,并且由于我们也在使用TF-IDF向量化,因此简单的点积将为我们提供相同的余弦相似度得分。现在什么是TF-IDF向量?我们无法以数据集中的形式计算给定描述之间的相似度。这实际上是不可能的。为此,将为所有文档计算术语频率-反文档频率(TF-IDF),这将简单地返回一个矩阵,每个单词代表一列。 sklearn的TfidfVectorizer将通过以下几行为我们做到这一点:
# In[2]:
# reading file
book_description = pd.read_csv('description.csv', encoding = 'latin-1')
# In[3]:
# checking if we have the right data
book_description.head()
# In[4]:
# removing the stop words
books_tfidf = TfidfVectorizer(stop_words='english')
# filling the missing values with empty string
book_description['description'] = book_description['description'].fillna('')
# computing TF-IDF matrix required for calculating cosine similarity
book_description_matrix = books_tfidf.fit_transform(book_description['description'])
# In[5]:
# Let's check the shape of computed matrix
book_description_matrix.shape
The above shape means that 4186 words are used to describe 143 books in our dataset. 上面的形状表示使用4186个单词来描述我们数据集中的143本书。
# computing cosine similarity matrix using linear_kernal of sklearn
cosine_similarity = linear_kernel(book_description_matrix, book_description_matrix)
# In[6]:
indices = pd.Series(book_description['name'].index)
# In[7]:
# Function to get the most similar books
def recommend(index, cosine_sim=cosine_similarity):
id = indices[index]
# Get the pairwsie similarity scores of all books compared to that book,
# sorting them and getting top 5
similarity_scores = list(enumerate(cosine_sim[id]))
similarity_scores = sorted(similarity_scores, key=lambda x: x[1], reverse=True)
similarity_scores = similarity_scores[1:6]
# Get the books index
books_index = [i[0] for i in similarity_scores]
# Return the top 5 most similar books using integer-location based indexing (iloc)
return book_description['name'].iloc[books_index]
# In[8]:
# getting recommendation for book at index 2
recommend(2)
# In[9]:
# getting recommendation for book at index 6
recommend(6)
If you notice the results we got; book at the index 2 is similar to book at index 6 according to our recommendation engine. Let’s follow along the description and see if our recommender is working. 如果您注意到我们得到的结果;根据我们的推荐引擎,索引2的书与索引6的书相似。让我们按照说明进行操作,看看我们的推荐人是否正在工作。
As per goodreads; here’s the very short description of the “Angela’s Ashes”: 根据读物;这是“安格拉的灰烬”的简短描述:
"When I look back on my childhood, I wonder how I managed to survive at all. It was, of course, a miserable childhood: the happy childhood is hardly worth your while. Worse than the ordinary miserable childhood is the miserable Irish childhood, and worse yet is the miserable Irish Catholic childhood." “当我回顾自己的童年时,我想知道我是如何做到生存的。这当然是一个悲惨的童年:快乐的童年几乎不值得你度过。比普通的悲惨的童年还差的是爱尔兰的悲惨的童年,更糟糕的是悲惨的爱尔兰天主教童年。”
And “Running with Scissors” goes as: 并且``用剪刀运行''的方式为:
“The true story of an outlaw childhood where rules were unheard of, the Christmas tree stayed up all year round, Valium was consumed like candy, and if things got dull, an electroshock-therapy machine could provide entertainment.” “一个真正的非法童年故事,规则从未被听见,圣诞树整年都呆着,Valium像糖果一样被消耗掉,如果事情变暗了,电击疗法机可以提供娱乐。”
Which shows somewhat similarity between the synopsis of the story. Also both books belong to the genre ‘Biographies & Memoirs’. This shows that our recommendation is good enough with all its simplicity. 这表明故事的提要之间有些相似之处。同样,这两本书都属于“传记与回忆录”类型。这表明我们的建议非常简单,足够好。